程序示例精选
Python+Yolov8路面桥梁墙体裂缝识别
如需安装运行环境或远程调试,见文章底部个人QQ名片,由专业技术人员远程协助!
前言
这篇博客针对《Python+Yolov8路面桥梁墙体裂缝识别》编写代码,代码整洁,规则,易读。 学习与应用推荐首选。
运行结果
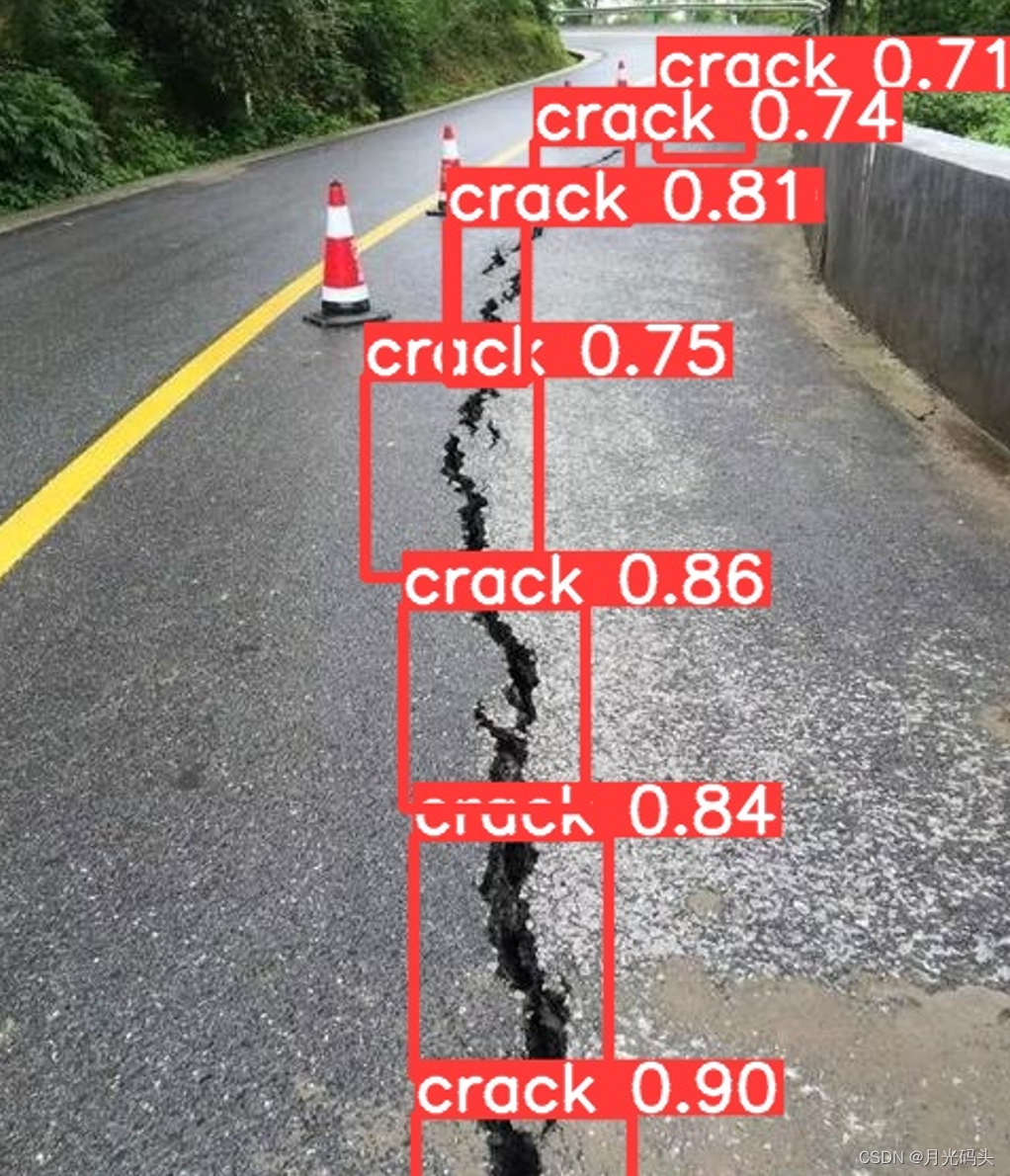
文章目录
一、所需工具软件
二、使用步骤
1. 主要代码
2. 运行结果
三、在线协助
一、所需工具软件
1. Python
2. Yolov8
二、使用步骤
代码如下(示例):
<code class="language-plaintext hljs">import torch
from ultralytics.yolo.engine.predictor import BasePredictor
from ultralytics.yolo.engine.results import Results
from ultralytics.yolo.utils import DEFAULT_CFG, ROOT, ops
from ultralytics.yolo.utils.plotting import Annotator, colors, save_one_box</code>
<code class="language-plaintext hljs">class DetectionPredictor(BasePredictor):
def get_annotator(self, img):
return Annotator(img, line_width=self.args.line_thickness, example=str(self.model.names))
def preprocess(self, img):
img = torch.from_numpy(img).to(self.model.device)
img = img.half() if self.model.fp16 else img.float() # uint8 to fp16/32
img /= 255 # 0 - 255 to 0.0 - 1.0
return img
def postprocess(self, preds, img, orig_img):
preds = ops.non_max_suppression(preds,
self.args.conf,
self.args.iou,
agnostic=self.args.agnostic_nms,
max_det=self.args.max_det,
classes=self.args.classes)
results = []
for i, pred in enumerate(preds):
orig_img = orig_img[i] if isinstance(orig_img, list) else orig_img
shape = orig_img.shape
pred[:, :4] = ops.scale_boxes(img.shape[2:], pred[:, :4], shape).round()
results.append(Results(boxes=pred, orig_img=orig_img, names=self.model.names))
return results
def write_results(self, idx, results, batch):
p, im, im0 = batch
log_string = ''
if len(im.shape) == 3:
im = im[None] # expand for batch dim
self.seen += 1
imc = im0.copy() if self.args.save_crop else im0
if self.source_type.webcam or self.source_type.from_img: # batch_size >= 1
log_string += f'{idx}: '
frame = self.dataset.count
else:
frame = getattr(self.dataset, 'frame', 0)
self.data_path = p
self.txt_path = str(self.save_dir / 'labels' / p.stem) + ('' if self.dataset.mode == 'image' else f'_{frame}')
log_string += '%gx%g ' % im.shape[2:] # print string
self.annotator = self.get_annotator(im0)
det = results[idx].boxes # TODO: make boxes inherit from tensors
if len(det) == 0:
return log_string
for c in det.cls.unique():
n = (det.cls == c).sum() # detections per class
log_string += f"{n} {self.model.names[int(c)]}{'s' * (n > 1)}, "
# write
for d in reversed(det):
cls, conf = d.cls.squeeze(), d.conf.squeeze()
if self.args.save_txt: # Write to file
line = (cls, *(d.xywhn.view(-1).tolist()), conf) \
if self.args.save_conf else (cls, *(d.xywhn.view(-1).tolist())) # label format
with open(f'{self.txt_path}.txt', 'a') as f:
f.write(('%g ' * len(line)).rstrip() % line + '\n')
if self.args.save or self.args.save_crop or self.args.show: # Add bbox to image
c = int(cls) # integer class
name = f'id:{int(d.id.item())} {self.model.names[c]}' if d.id is not None else self.model.names[c]
label = None if self.args.hide_labels else (name if self.args.hide_conf else f'{name} {conf:.2f}')
self.annotator.box_label(d.xyxy.squeeze(), label, color=colors(c, True))
if self.args.save_crop:
save_one_box(d.xyxy,
imc,
file=self.save_dir / 'crops' / self.model.model.names[c] / f'{self.data_path.stem}.jpg',
BGR=True)
return log_string</code>
<code class="language-plaintext hljs">if __name__ == '__main__':
parser = argparse.ArgumentParser()
parser.add_argument('--weights', nargs='+', type=str, default='yolov5_best_road_crack_recog.pt', help='model.pt path(s)')
parser.add_argument('--img-size', type=int, default=640, help='inference size (pixels)')
parser.add_argument('--conf-thres', type=float, default=0.25, help='object confidence threshold')
parser.add_argument('--iou-thres', type=float, default=0.45, help='IOU threshold for NMS')
parser.add_argument('--view-img', action='store_true', help='display results')
parser.add_argument('--save-txt', action='store_true', help='save results to *.txt')
parser.add_argument('--classes', nargs='+', type=int, default='0', help='filter by class: --class 0, or --class 0 2 3')
parser.add_argument('--agnostic-nms', action='store_true', help='class-agnostic NMS')
parser.add_argument('--augment', action='store_true', help='augmented inference')
parser.add_argument('--update', action='store_true', help='update all models')
parser.add_argument('--project', default='runs/detect', help='save results to project/name')
parser.add_argument('--name', default='exp', help='save results to project/name')
parser.add_argument('--exist-ok', action='store_true', help='existing project/name ok, do not increment')
opt = parser.parse_args()
</code>
运行结果
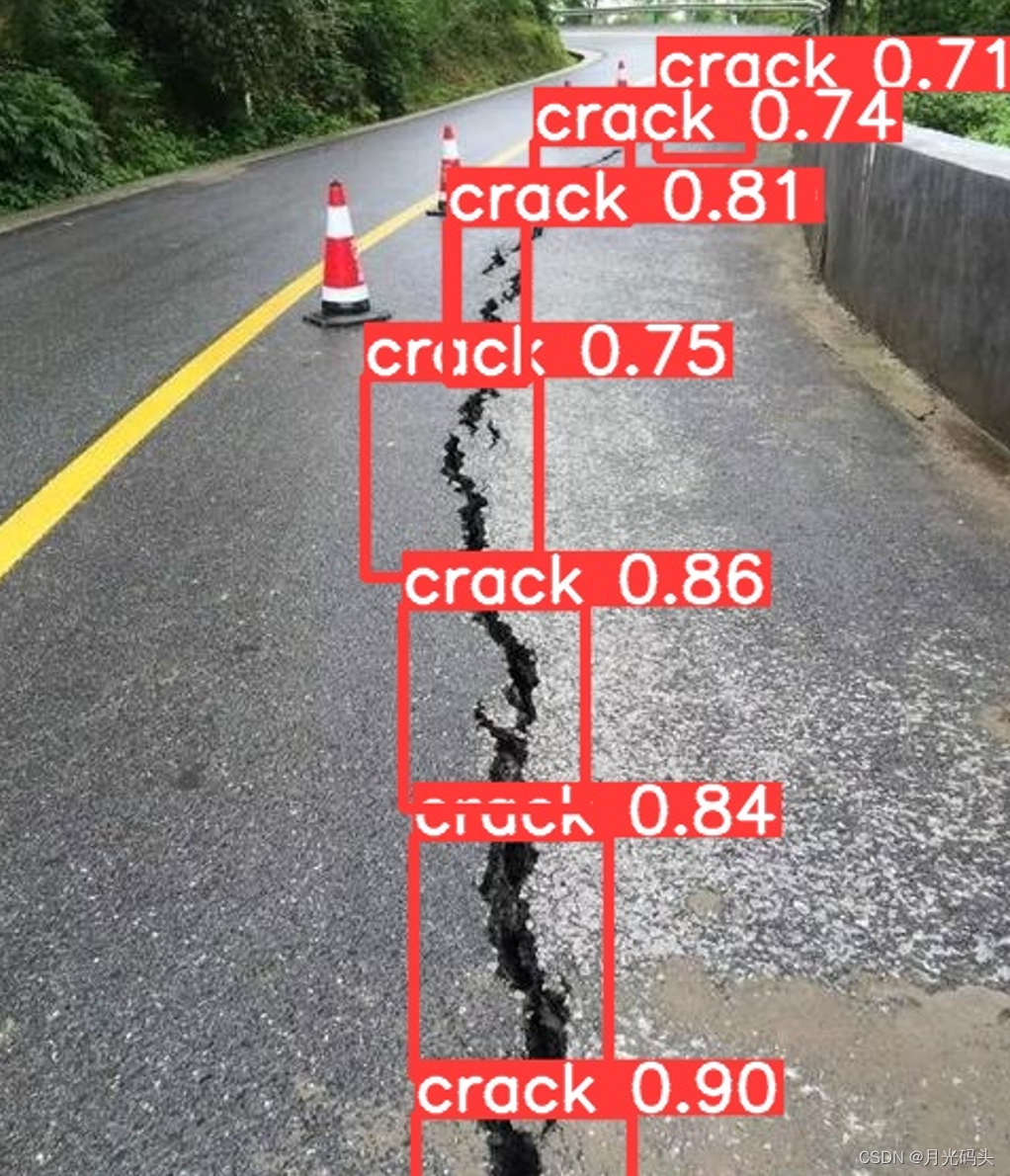
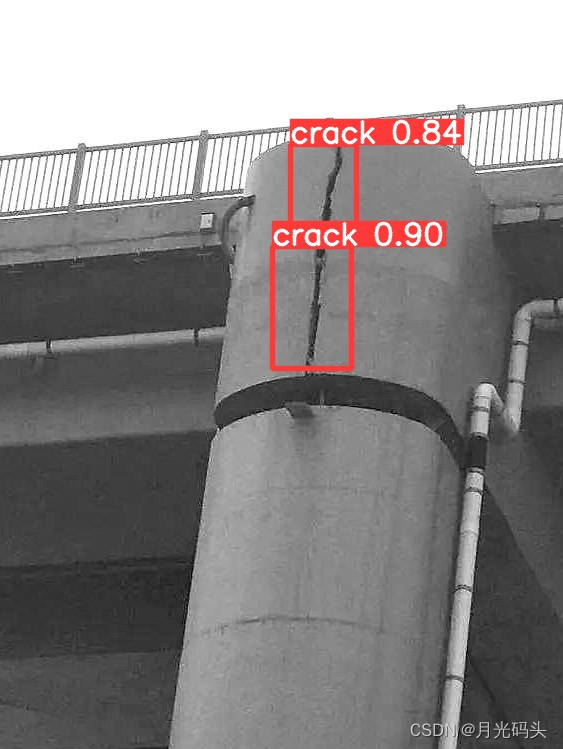
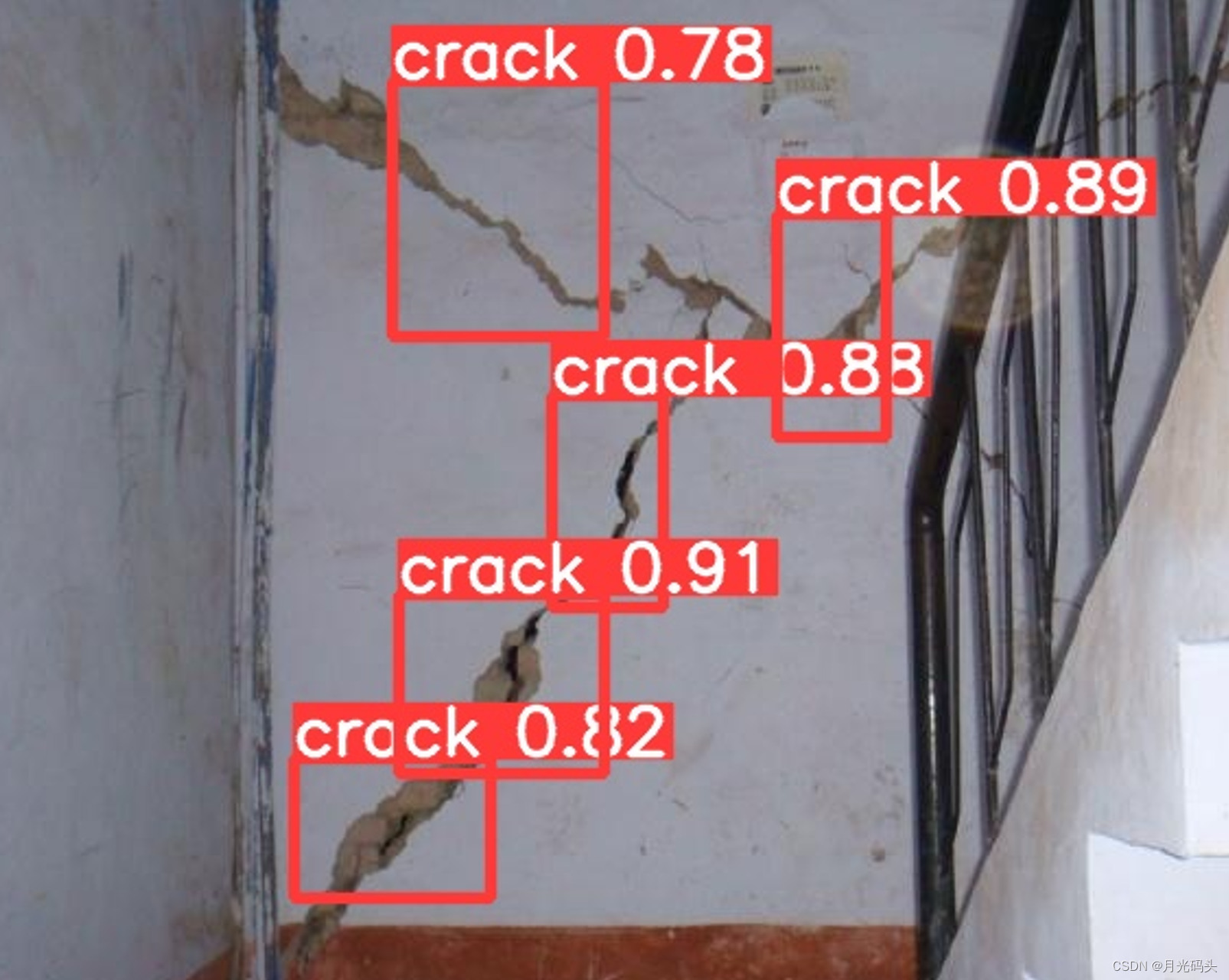
三、在线协助:
如需安装运行环境或远程调试,见文章底部个人 QQ 名片,由专业技术人员远程协助!
1)远程安装运行环境,代码调试
2)Visual Studio, Qt, C++, Python编程语言入门指导
3)界面美化
4)软件制作
当前文章连接:https://blog.csdn.net/alicema1111/article/details/132666851
个人博客主页:https://blog.csdn.net/alicema1111?type=blog
博主所有文章点这里:https://blog.csdn.net/alicema1111?type=blog